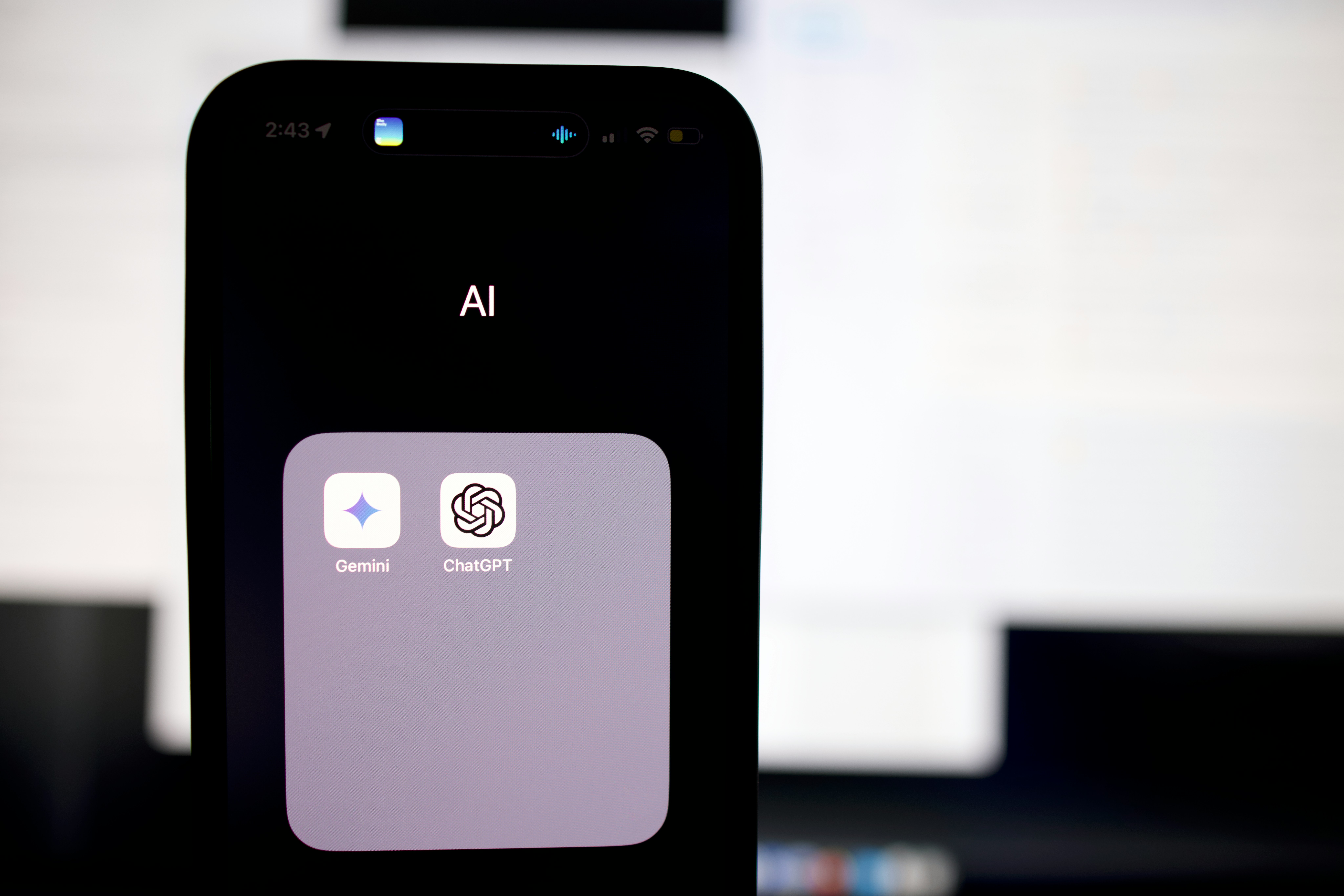
Nov 1, 2024
💡 This article is a follow-up to "The Rise of On-Device AI: Challenges and Solutions" Reading the previous article will help you better understand this content.
AI services like ChatGPT, Claude, and Perplexity have become deeply integrated into our daily lives. Many companies are either developing their own AI or leveraging generative AI to innovate their operations. Today, we'll explore the challenges faced by companies building AI services.
The reality of the AI industry is more challenging than expected. According to a recent report by the RAND Corporation, over 80% of AI projects fail - twice the failure rate of general IT projects. Based on interviews with 65 data scientists and engineers, the report analyzed the main causes of AI project failures. Let's examine these through three key aspects.
Human Factor: Misaligned Expectations and Understanding
Most project failures begin with industry stakeholders misunderstanding or miscommunicating the problem. Many AI companies view AI as a universal solution, but reality differs. AI has limited scope and more constraints than anticipated.
Successful AI projects require two types of expertise: deep understanding of the target industry and technical knowledge of AI technology. Finding the right balance between these two is crucial for setting realistic goals.
Environmental Challenges: Real-World Constraints
Not all AI operates in stable network environments like ChatGPT or Claude. Real-world applications face unstable networks, strict privacy requirements, real-time processing needs, and various edge devices. Traditional cloud-based AI often struggles under these conditions.
On-device AI can address these environmental challenges. By running AI directly on devices without network connectivity, it can solve network stability and security issues. However, limitations exist depending on device performance. While mobile NPU chip performance has improved significantly, challenges remain for other types of devices.
Cost Issues: Growing Burden
The AI industry faces a significant irony: while most services are offered free to users, providers bear enormous costs. For example, a voice recognition AI app with 10 million users can incur annual server costs of $3M, which increase exponentially with user growth.
More concerning is the unpredictability of costs due to rapid industry changes. Limited revenue coupled with unpredictable costs creates a ticking time bomb scenario.
On-device AI offers an innovative solution here. By running AI directly on devices without cloud infrastructure, it can dramatically reduce server costs. In an AI service market that has become a chicken game, this represents more than just cost reduction - it's a disruptive innovation.
Conclusion:
Emergence of a New Solution The three challenges we've examined - human, environmental, and cost factors - are closely interconnected, often creating a domino effect where one problem exacerbates another. The 80% failure rate of AI projects indicates the need for a new approach.
On-device AI presents an interesting alternative, offering several advantages:
Stable service delivery without network dependency
Enhanced data security and privacy protection
Reduced cloud server costs
Real-time processing capability
The potential for on-device AI is expanding, particularly with recent improvements in mobile device NPU (Neural Processing Unit) performance. Like Tesla's autonomous driving or Apple's Siri, which already process much AI directly on devices, on-device AI can be highly effective in specific domains.
However, on-device AI isn't a universal solution for all AI projects. Limitations exist, such as device performance constraints and complex computational requirements. Yet, in appropriate use cases, it can provide a cost-effective and stable solution, potentially increasing the success rate of AI projects.
As AI technology continues to advance and penetrate various industries, the importance of such solutions will likely grow. For successful AI projects, it's crucial to accurately assess project characteristics and requirements, choosing the most appropriate approach for each specific case.